Researcher Spotlight #42: Nathanael Larigaldie
A short talk with Nathanael about his research and interests.
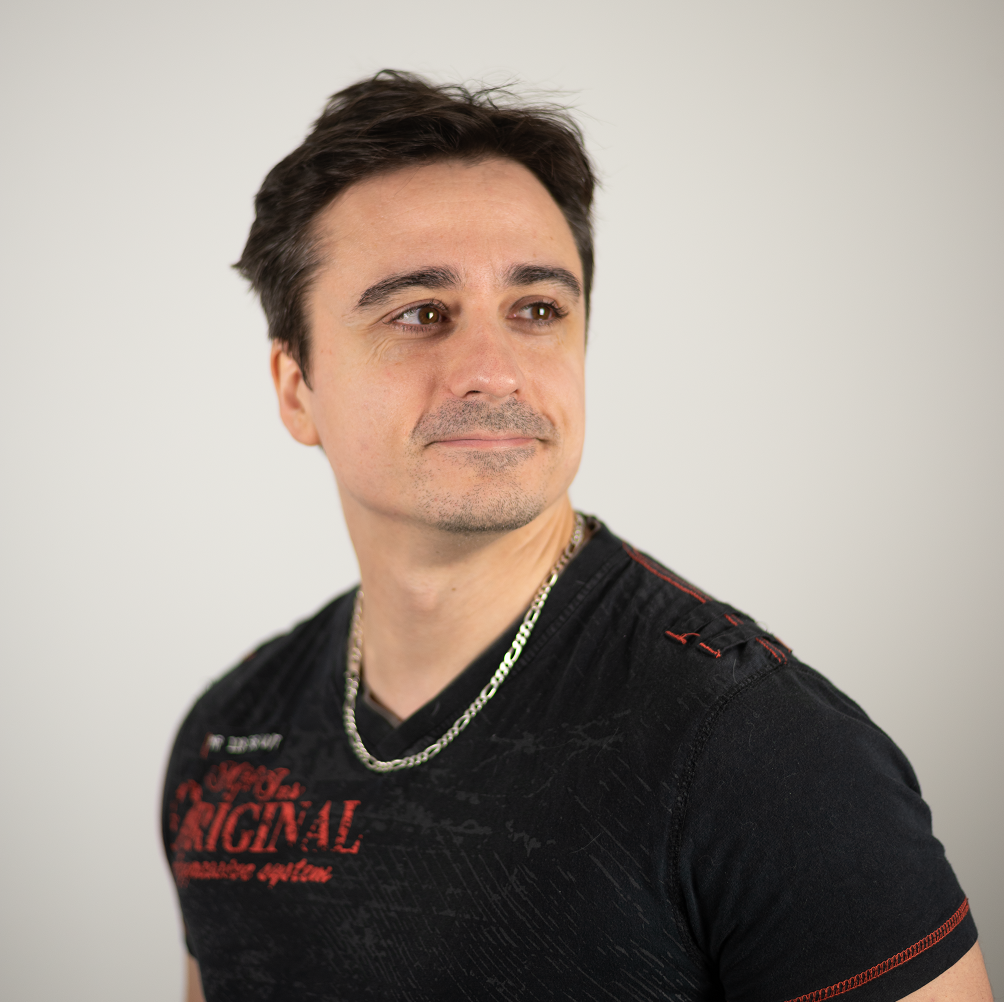
Hi Nathanael,
What is your background?
I am originally from France, but have moved around quite a bit. I did my Bachelor's degree in Poitiers (France), my Master's degree in Louvain-la-Neuve (Belgium) and my PhD in Durham (UK), all in Psychology. Officially, that qualifies me as a Psychology researcher, but I have also studied Computer Science and have worked as a software developer both freelance and in industry. In fact, I am happiest when I can apply computational methods to my research projects.
What are your main areas of research, and what do you think makes them interesting and worth studying? Do you have any collaborators?
That's a tough one because it's constantly changing! In general, I am more interested in the methodological and technical aspects of research, so I prefer to select projects based on the methods that interest me. However, cognitive science has always served as the foundation for my projects, and I am naturally drawn to metascience research.
My current research project, in collaboration with Jacob L. Orquin and Sonja Perkovic, seeks to investigate the relationship between visual attention and attitudes towards real-life argumentation found, say, on social media. This could allow us to draw conclusions and gain insights into online political polarization, which many see as a significant threat to modern democracies.
To achieve a sufficient number of trials and participants for this project, we also had to create (in collaboration with Anna Dreneva) a new computational method to address a long-standing problem in eye tracking research: mapping eye tracker coordinates to overflowing/scrollable content. This resulted in the creation of the R package eyeScrollR, which we hope to see used in many projects around the world!
How did you hear about Cognition and Behavior Lab?
COBE Lab is the go-to place for my research group's study setup and participant recruitment, so I heard about it as soon as I started working at my current department.
How have you used the lab so far? What are the main benefits of being able to use the lab in your opinion?
I've already used the Lab to conduct several correlational and experimental studies using different eye trackers (EyeLink and Aurora), as well as a software validation study.
After visiting several labs around the world, I can attest that COBE Lab is a breeze to work with in comparison. The ethics application process is straightforward and quick, and the participant management and recruitment system couldn't be simpler. A big shout out to Marc and Dan for keeping such a well-organized lab; and yes, the awesome coffee is definitely a nice touch!
Is there a particular recommendation you would like to pass on to other researchers? Something you wish you had known before you started, or just a useful trick.
If you work in a quantitative field, you should prioritize early and consistent training in programming, statistics, and, most importantly, open science principles. In the last 20 years, metascience research has consistently shown that many fields have significant problems that researchers can no longer ignore, but our formal training on best scientific practices remains largely inadequate. We owe it to other researchers, funding sources, the general public, and our own intellectual integrity to be transparent about our data, methods, and thinking processes. I do not believe there is a way to address the current issues without embracing this.
Could you recommend one academic book to fellow colleagues that you think is of great interest?
I am torn between the great and ever-improving Improving Your Statistical Inferences by Daniël Lakens (available online for free!) and the brilliant Statistical Rethinking by Richard McElreath. But since I have to choose one, I'll go with the latter.
Statistical rethinking is an excellent applied introduction to Bayesian statistics and the often-overlooked theme of causal inference, but it is much more than that, and even those who prefer to stick with traditional frequentist approaches will benefit greatly from the reading. The book provides a thorough understanding of the inner mechanisms of statistical inference and GLMM while remaining accessible to scientists with a light background in mathematics and programming (though prior knowledge of applied statistics is recommended). Most importantly, the author tightly connects statistical analyses with scientific model development, which is how it should be but unfortunately rarely is. If nothing else, I think all quantitative scientists should at the very least read and reflect on the first chapter.
Finally, what do you enjoy doing away from your research?
Programming has been one of my hobbies for a long time, as have video games; for all fellow nerds out there, I began my gaming journey on an Amstrad CPC 6128 when I was 6. Otherwise, drumming would take the cake if I had more time to devote to it. I still can't decide whether I want to be an academic or a rock star when I grow up.